Computational biology
The Computational Biology group addresses biological problems using computational methods, focusing on mitochondria as important metabolic organelles and on the evolution of biological systems.
Mitochondrial computational biology: One of our research interests is to understand mitochondrial heterogeneity and diversity of structure, function and expression dynamics across different tissues, but also in different disease conditions. We use big data and big data integration techniques to learn how mitochondria adapt to their cellular environment. Our data visualization and integration platform mitoXplorer (http://mitoxplorer2.ibdm.univ-mrs.fr) helps us use omics data to investigate this mitochondrial behaviour.
Network biology: In a second project, we use complex networks to investigate the temporal behaviour of biological systems – monitored by temporal protein or RNA expression dynamics. With the help of these techniques, we can also unravel different phases of mitochondrial expression dynamics in development, ageing or in disease progression.
Evolutionary computational biology: We are also interested in evolutionary computational biology on sequence, cellular, and organismal level. We currently have several projects related to evolutionary computational biology. On sequence level, we are investigating the evolution of short linear motifs in proteins (SLiMs) and look specifically at predatory, as well as mechano-sensing motifs (in collaboration with the team of Tam Mignot). On a cellular level, we look at the evolution of epithelia in the most primitive metazoan organisms (sea-water sponges) as an important structure to define organismal and tissue boundaries (in collaboration with Andre le Bivic and Carole Borchiellini). On an organismal level, we look at the evolution of predatory traits in bacteria (in collaboration with the lab of Tam Mignot).
Computational tool development for biological data mining and integration: Our lab is developing user-friendly tools for general data analysis, data mining and data integration for the research community. Find out here what these are and where to find them.
Publications
The mitoXplorer 2.0 update: integrating and interpreting mitochondrial expression dynamics within a cellular context
Evolution of mechanisms controlling epithelial morphogenesis across animals: new insights from dissociation-reaggregation experiments in the sponge Oscarella lobularis
AnnoMiner is a new web-tool to integrate epigenetics, transcription factor occupancy and transcriptomics data to predict transcriptional regulators
RNfuzzyApp: an R shiny RNA-seq data analysis app for visualisation, differential expression analysis, time-series clustering and enrichment analysis
Introducing the novel Cytoscape app TimeNexus to analyze time-series data using temporal MultiLayer Networks (tMLNs)
Inferring cell cycle phases from a partially temporal network of protein interactions
The mitoXplorer 2.0 update: integrating and interpreting mitochondrial expression dynamics within a cellular context
Evolution of mechanisms controlling epithelial morphogenesis across animals: new insights from dissociation-reaggregation experiments in the sponge Oscarella lobularis
AnnoMiner is a new web-tool to integrate epigenetics, transcription factor occupancy and transcriptomics data to predict transcriptional regulators
RNfuzzyApp: an R shiny RNA-seq data analysis app for visualisation, differential expression analysis, time-series clustering and enrichment analysis
Introducing the novel Cytoscape app TimeNexus to analyze time-series data using temporal MultiLayer Networks (tMLNs)
Evaluating the landscape of gene cooperativity with receptor tyrosine kinases in liver tumorigenesis using transposon-mediated mutagenesis
Phenotypic and genomic comparison of Photorhabdus luminescens subsp. laumondii TT01 and a widely used rifampicin-resistant Photorhabdus luminescens laboratory strain
Hypermethylation of gene body CpG islands predicts high dosage of functional oncogenes in liver cancer
A transcriptomics resource reveals a transcriptional transition during ordered sarcomere morphogenesis in flight muscle.
Integrative analysis and machine learning on cancer genomics data using the Cancer Systems Biology Database (CancerSysDB).
The deregulated microRNAome contributes to the cellular response to aneuploidy.
SLALOM, a flexible method for the identification and statistical analysis of overlapping continuous sequence elements in sequence- and time-series data
The axolotl genome and the evolution of key tissue formation regulators.
High-resolution TADs reveal DNA sequences underlying genome organization in flies.
HH-MOTiF: de novo detection of short linear motifs in proteins by Hidden Markov Model comparisons
Revision and reannotation of the Halomonas elongata DSM 2581T genome.
A Guide to Computational Methods for Predicting Mitochondrial Localization.
Oh Brother, Where Art Thou? Finding Orthologs in the Twilight and Midnight Zones of Sequence Similarity
Structure of a Cytoplasmic 11-Subunit RNA Exosome Complex.
Secretory cargo sorting by Ca2+-dependent Cab45 oligomerization at the trans-Golgi network.
Human Holliday junction resolvase GEN1 uses a chromodomain for efficient DNA recognition and cleavage.
Virtual pathway explorer (viPEr) and pathway enrichment analysis tool (PEANuT): creating and analyzing focus networks to identify cross-talk between molecules and pathways.
Tools for visualization and analysis of molecular networks, pathways, and -omics data.
The RNA-binding protein Arrest (Bruno) regulates alternative splicing to enable myofibril maturation in Drosophila flight muscle.
Merging and scoring molecular interactions utilising existing community standards: tools, use-cases and a case study.
morFeus: a web-based program to detect remotely conserved orthologs using symmetrical best hits and orthology network scoring.
PsicquicGraph, a BioJS component to visualize molecular interactions from PSICQUIC servers.
KEGGViewer, a BioJS component to visualize KEGG Pathways.
MTERF1 binds mtDNA to prevent transcriptional interference at the light-strand promoter but is dispensable for rRNA gene transcription regulation.
Designing efficient and specific endoribonuclease-prepared siRNAs.
HMMerThread: detecting remote, functional conserved domains in entire genomes by combining relaxed sequence-database searches with fold recognition.
SeLOX–a locus of recombination site search tool for the detection and directed evolution of site-specific recombination systems.
Genome-wide resources of endoribonuclease-prepared short interfering RNAs for specific loss-of-function studies.
ProFAT: a web-based tool for the functional annotation of protein sequences.
An Ambystoma mexicanum EST sequencing project: analysis of 17,352 expressed sequence tags from embryonic and regenerating blastema cDNA libraries.
DEQOR: a web-based tool for the design and quality control of siRNAs.
The power and the limitations of cross-species protein identification by mass spectrometry-driven sequence similarity searches.
News
We’re pleased to share some great news about our researchers’ achievements! Several projects from our teams have been selected for funding by the ANR and FRM, highlighting their hard work and innovative research.
Congratulations to Robert Kelly, Frank Schnorrer, Cédric Maurange, Bianca Habermann and Delphine Delacour!
IBDM Marseille inspires young minds: engaging primary school children on childhood cancer (“Contre le cancer, j’apporte ma pierre”) and interacting with high school students through immersive experiences (DECLICS).
Join us on 29/06/2023 at 12:30 in Amphi 12 for an exciting talk by Rikesh Jain and Theo Brunet from our Team!
5 motivated and talented students successfully defended their thesis between September 2022 and January 2023.
Show me your rhythm!
We introduce an algorithm, Phasik, for extracting the phases of biological systems by clustering partial temporal networks.
Self-organisation of human muscles in a dish
Human muscle cells self-organise into defined fiber bundles in vitro even without the presence of external cues !
We introduce a novel, user-friendly web-based tool ‘AnnoMiner’ to annotate and integrate epigenetic and transcription factor binding data.
Look at the TIME in your interaction network
The Habermann team has repurposed the concept of multilayer networks generally used to integrate different types of data.
Our team at the IBDM is part of a Maitre de Conference competition for a permanent assistant professor position in Bioinformatics in Aix-Marseille University.
Team members
Alumni
Funding bodies
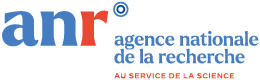
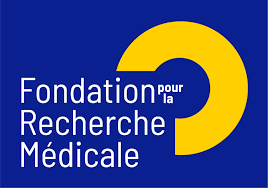
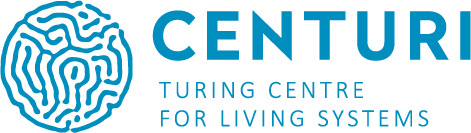